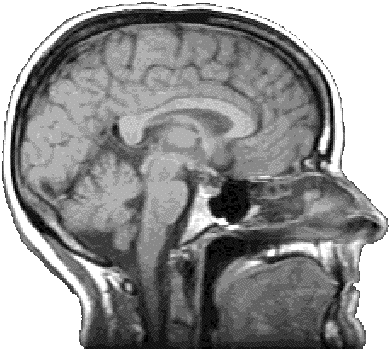
|
- Derive the origin of emotion based on first principles
in evolution theoretically
- Develop a comprehensive model of emotional processing
in autonomous being (animals and autonomous robots)
called EMOTION (Emotional Model of The Theoretical
Interpretation of Neuroprocessing
- » EMOTION-I model: accounts for "emotional
feel" in sensations in evolution
- » EMOTION-II model: accounts
for emergence of happy and unhappy emotions in evolution
- Reveal the role of emotions in cognitive processing
in neural decision making processes
- Confirm the EMOTION models experimentally in human
- Develop statistical techniques to analyze multiple
spike train signals
- Derive the principles of spike train interactions
among neurons in a neural network
- Correlate the spike train signals with physiological
and cognitive functions to deduce their signal contents
- Extract neural spike code signal from extraceullar
recordings of action potentials
- Reverse-engineer the principles of operations in
neural processing
- Decode the neural signals used by neurons in a neural
network
- Solve the encoding/decoding problems of repesentation
of signals by pulse-coded signal
- Abstract the low-level spike train signals into higher-level
representation of message content
- Develop a simulation model for reconstructing the
neuroelectrophysiological functions of a neuron called
MacNeuron.
- Develop a simulation model of neural network with
auto-associative reinforcement learning
- Implement the generalizeable, scaleable principles
for building a computational model of the brain
- Implement the object-oriented principles for building
an extensible model of the brain
- Integrate analytical model in the neural simulators
- Develop efficient representation of neural signals
for predictive control in neuro-assisted prosthetic
devices
- Implement the encoding and decoding schemes of neural
representation for neuroprosthetic control
- Develop self-adaptive, predictive control for error-correcting
neuroprosthetic motor control
- Develop brain-computer interface (BCI) to use brain-controlled commands for robotics control
- Investigate the principles for self-actuating, autonomous
systems
- Apply the neural network learning algorithms in autonomous
robot control
- Investigate the real-world feedback interactions
for evolving controling functions
- Translate the principles between autonomous robots
and self-actuating biological organisms
- Brain imaging using non-invasive optical signals that penetrate brain tissues
- Optical imaging of the brain using functional near-infrared spectroscopy
- Record cortical brain activations based on oxy- and deoxy-hemoglobin optical signals
- Correlation of cognitive activities with hemodynamics in localized cortical areas
- Imaging of motor cortical activities to decode intentional movement directions
- Effects of physical exercise (aerobic activities) on improvement of cognitive performance
- Identify specific cognitive enhancements from specific motivated/ skilled aerobic exercises
- Identify the neural correlates of mind-body connection between physical and mental activities
- Derive the principles and criteria for consolidating
learned functions into the next generation
- Explore the mechanisms for transfer from learned
circuitry to reflex control circuitry
- Implement a neural network model for evolutionary
learning that spans multiple generations
|